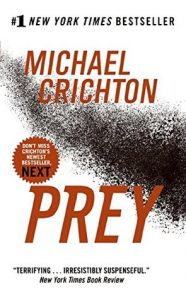
A cover of Michael Crichton’s Prey.
Every so often, I’ll get the question, ‘So, have you ever found any dinosaurs?’ Although I’d honestly love to encounter a dinosaur bone or two on an archaeological excavation, as a student of archaeology I have to respond that dinosaurs are generally the realm of the palaeontologist, and what I deal with are the remains of human activity. Although… Lately, I’ve been reading a book by Michael Crichton, the author of the classic dino-thriller Jurassic Park. It seems that the man who popularised dinosaurs in modern media also had ambitions for the future of Roman pottery study. The Crichton novel I’m talking about is titled Prey, and within the text is the following passage:
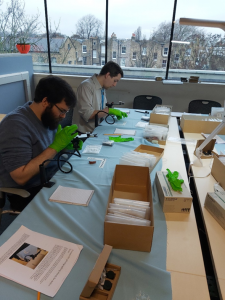
The Arch-I-Scan team is in the early phases of data collection, taking hundreds of thousands of photos of Roman fine ware pottery in repositories all over England.
“The swarm represented an extremely high-resolution camera combined with a distributed intelligence network. And one thing distributed networks did particularly well was detect patterns. That was why distributed network programs were used to recognize faces for security systems, or to assemble the shattered fragments of archaeological pottery. The networks could find patterns in data better than the human eye.”
Published nearly two decades ago in 2002, the book tackles the increasingly popular topic of artificial intelligence. Some of the science-fiction he imagined then has since come into reality and common use. Most people today are well aware of the technological breakthroughs in facial recognition. It’s taken a bit longer for this artificial intelligence technology to take off in the world of archaeology, yet this is precisely the topic being explored by the University of Leicester School of Archaeology and Ancient History’s Arch-I-Scan project.
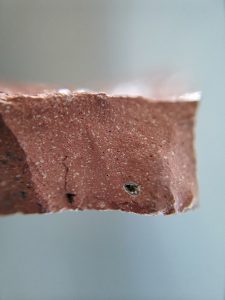
An example of a fresh break in a Roman pot sherd.
Currently, in the world of archaeology, the task of identifying pottery for publication is largely the province of pottery specialists who identify samples on the basis of form (shape of vessel) and fabric (the makeup of the internal clay, which can be seen after having made a fresh break in the pottery sample). It takes years of training to reach such a level of expertise. In Prey, Michael Crichton envisaged that ‘The networks could find patterns in data better than the human eye.’ Just as Crichton suggested, artificial intelligence technology now has the potential to analyse images and identify thousands of features beyond what we as humans may see and use as identification metrics. Each year, the ImageNet Large Scale Visual Recognition Challenge (ILSVRC) takes place, in which computer technology is pitted against humans in image recognition challenges. The graphic below shows some results of this competition related to percentage error of identification: the human eye was bettered by the the AI in 2015, and the technology only continues to get better.
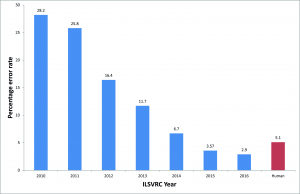
Results of the ILSVRC from 2010-2016. From Tyukin et. al. 2018. Data from ImageNet; Karpathy 2014.
An archaeologist will identify a piece of pottery as a certain ‘type; according to a number of observable categories, including colour, texture, size, shape, curvature, wall thickness, presence or absence of specific diagnostic features, etc.). When an AI service ‘reads’ a photo of the same piece of pottery, it may identify thousands of additional features according to different sections of the photo, and if it compares these features to what it has already learned from previous photos or input data, the AI machine-learning service can, in theory, isolate patterns and identify better than the human eye. Such a service would aid in the quick and consistent identification of pottery samples. Now, Arch-I-Scan is working to turn that theory into archaeological practice.
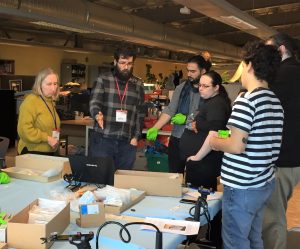
Santos, our Maths postdoctoral research associate, explains machine-learning to volunteers at the Museum of London Archaeology.
Indeed, it’s true that an AI service can process images and hone in on large numbers of features relatively quickly, but it can only convert these feature-patterns into useful object identifications if the AI service has a large enough knowledge-base. AI image recognition operates similarly to the ways in which humans identify new things that they see: comparison of the new object to what is recognised from prior experience. After it has been trained to a degree, the machine should become more consistent in its recognition, or free from what pottery specialists know to be errors. The AI will become more intelligent according to the premise the more it sees, the more it learns. This self-learning component is crucial, but it can also be one of the most confusing or frustrating aspects of the research. The computer will generate rules based on raw data that has been fed into it, but we don’t necessarily know what rules it is making up for itself to isolate patterns in the data. Instead, we ‘train’ the machine by feeding it hundreds of thousands of varied, real-life images from which to learn. There are certain tricks our mathematicians may use to guide the AI machine on its learning journey, but for now the Arch-I-Scan team must collect as many photos of Roman ceramic table wares as possible in collaboration with our project partners: the Museum of London, Museum of London Archaeology, University of Leicester Archaeological Services, Vindolanda Charitable Trust, and Colchester and Ipswich Museum Service.
Prey, like Jurassic Park, is a cautionary tale about the ethics of exploring new technologies, and whether or not we should enter that brave new world, even if we can. It may then be worth highlighting that the aim is not to eliminate the need for pottery specialists. Rather, a successful machine-learning service would speed up post-excavation processing, allowing specialists to perform more comprehensive studies, the likes of which can often be impeded by time and budget constraints. Furthermore, it may facilitate pottery identification for various non-specialists including museum professionals, scholars, and interested members of the public, ultimately fostering a greater engagement with this subset of material culture. Any such automated tools which promote quicker and more consistent identification would furthermore help to face the ‘Big Data Challenge’ in the world of cultural heritage – expanding the study the vast amount of legacy data which has built up over time.
Recent Comments